COMMERCE 2QA3 Study Guide - Final Guide: Subset, Categorical Variable, Collinearity
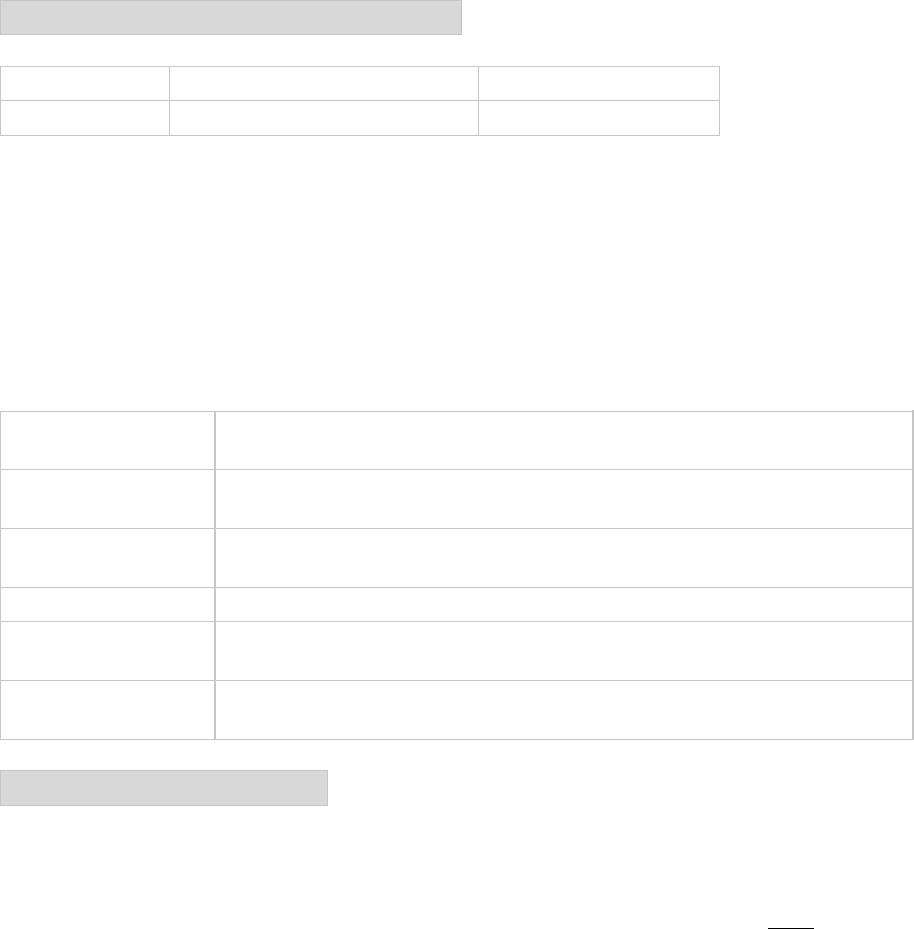
Model one variable in terms of multiple, other variables.
The Linear Multiple Regression Model
Simple regression
Only one predictor variable
Multiple regression
Multiple predictor variables
Residuals are still modelled by
.
Degrees of freedom are modelled by , where is the number of observations, and is the
number of predictor variables.
The standard deviation of the residuals is therefore
.
Interpreting Multiple Regression Coefficients
The meaning of the coefficients in multiple regression can be different than in simple regression. They must be
interpreted in terms of the other predictors in the model.
Assumptions
Linearity Assumption
Check the scatterplots for each predictor value and the residuals plot. Consider data
re-expression if necessary.
Independence
Assumption
There is no way to be sure the assumption is satisfied, so think about how the data
was collected to decide if the assumption is reasonable.
Equal Variance
Assumption
Variability of the errors should be similar for each predictor - use scatterplots to
assess.
Normality
Check to see if distribution of residuals is unimodal and symmetric.
Randomization
Condition
Does the collection method introduce any bias?
Check that x are linearly
related to y
Should NOT be linearly associated
Test the significance level of the model.
There are several hypothesis tests in multiple regression, each concerned with whether the underlying
parameters are actually zero.
Testing the Multiple Regression Model
= number of predictors
•
, where is the number of observations
•
The Null Hypothesis for slope coefficients is , and should be tested with an F-test, a
generalization of the t-test to more than one predictor variable. There are two degrees of freedom:
The F-test is one-sided, so bigger F-values mean smaller P-values. If the null hypothesis is true, F is near 1.
If it leads to the rejection of the null hypothesis, check the t-test statistic for each coefficient
.
Confidence interval is
.
The coefficient can be different from zero even when there is no correlation between y and and it's
even possible that the new regression slope changes signs when a new variable enters the regression
•
The meaning of the coefficient depends on the other predictors in the model. If we fail to reject based
on its t=test, it does not mean that has no linear relationship to y, but that contributes nothing to modeling
y after allowing for other predictors.
The F-Statistic and ANOVA
is the fraction of the total variation in y accounted for by the model, all predictor variables included, and is
represented by
. It can be shown that
, so testing if is
equivalent to testing if .
Multiple Regression
November 16, 2017
4:34 PM
Statistics Page 1
Document Summary
Model one variable in terms of multiple, other variables. Degrees of freedom are modelled by , where is the number of observations, and is the. The standard deviation of the residuals is therefore number of predictor variables. The meaning of the coefficients in multiple regression can be different than in simple regression. They must be interpreted in terms of the other predictors in the model. Check the scatterplots for each predictor value and the residuals plot. There is no way to be sure the assumption is satisfied, so think about how the data was collected to decide if the assumption is reasonable. Variability of the errors should be similar for each predictor - use scatterplots to assess. Check to see if distribution of residuals is unimodal and symmetric. Check that x are linearly related to y. There are several hypothesis tests in multiple regression, each concerned with whether the underlying parameters are actually zero.