DATA1001 Study Guide - Final Guide: Bayesian Probability, Marginal Distribution, Sample Space
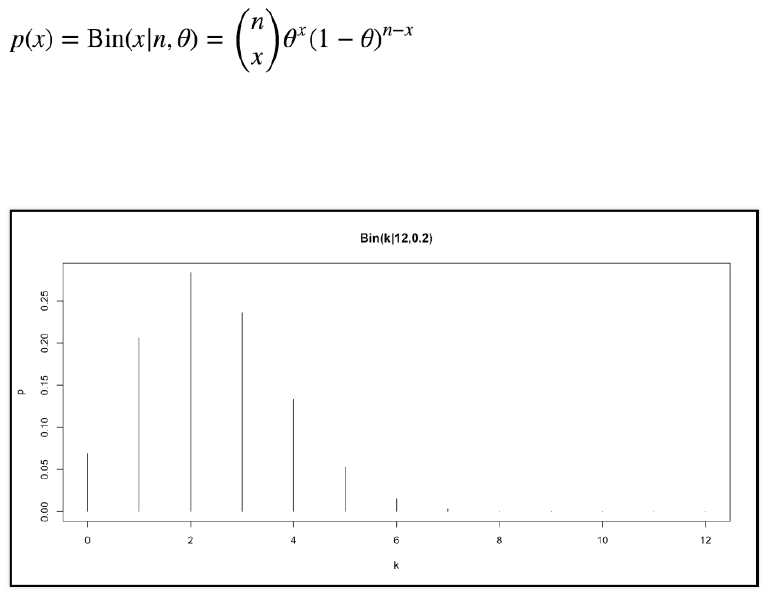
DATA1001¬es&&
What&is&probability?&
The&concept&is&the&same&as&that&of&a&“mass&distribution”&in&physics&(with&total&mass&1):&
• Assume&a&sample'space&(usually&Ω)&of&all#possible#outcomes.&(E.g.&coin&tosses;&stock&
market&curves;&number&of&customers&signing&up;&order&in&which&students&enter&the&
room;&…)&
• A&probability'distribution&(usually&p())&distributes&the&mass&1&over&the&sample&space.&
• An&event&(e.g.&A⊂Ω)&is&a&subset#of#the#sample#space.&
• The&mass&of&an&event&p(A)&is&called&its&probability.&
&
What&is&a&Random&Variable?&
There&is&a&one-to-one&correspondence&between&probability&distributions&and&random&
variables:&
• A&random&variable&X&can&take&any&value&in&the&sample'space.&
• The&probability&that&X&takes&a&value&from&the&set&A&is&p(A).&
The&two&points&show&how&any&probability&distribution&“defines”&a&random&variable&X.&(This&is&
made&rigorous&in&MATH5825.)&
&
Discrete&probability&distributions&
If&the&sample&space&can&be&written&as&a&(possibly&infinite)&list,&then&the&distribution&is&
called&discrete:&
Binomial&distribution:&x∼Bin(n,θ)&and&write&p(x)=Bin(x|n,θ)&
• parameters:&number&n&of&trials;&probability&θ&of&success&
• sample&space:&x∈{0,1,…,n}&
• distribution:&
&
&
(Note&that&Bin(x|1,θ)&is&also&called&the&“Bernoulli”&distribution,&and&written&as&Bern(x|θ).&A&
single&fair&coin&toss&corresponds&to&Bern(x|0.5).)&
&
Binomial&distribution&
&
&
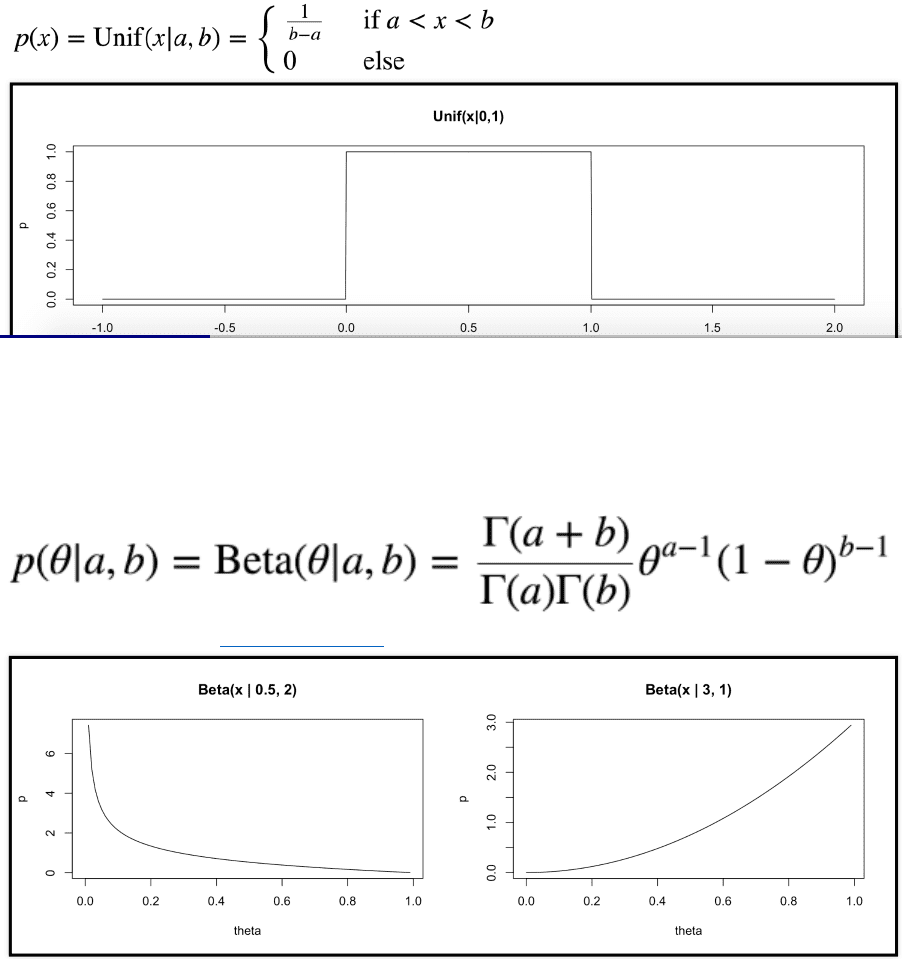
Continuous&probability&distributions&
If&it&is¬&possible&to&write&the&sample&space&as&a&list,&then&the&distribution&is&continuous.&
E.g.,&there&exists&no&(not&even&infinite)&list&of&all&real&numbers&between&0&and&1.&
We&use&the¬ation&p()&for&both&discrete&and&continuous&distribution.&But¬e:&
• If&p&is&discrete,&then&p(x)&is&a&probability&(think&“mass”).&
• If&p&is&continuous,&then&p(x)&is&a&probability&density&(think&“mass&per&
length/area/volume”).&
&
Uniform&distribution&
X∼Unif(a,b)&
• parameters:&a&(start)&and&b&(end)&
• sample&space:&the&interval&[a,b][a,b]&
• probability&density:&
&
&
&
Beta&distribution&
θ∼Beta(a,b)θ∼Beta(a,b)&
• parameters:&aa&and&bb&
• sample&space:&the&unit&interval&(0,1)(0,1)&
• probability&density:&
&
where&Γ()&denotes&the&Gamma&function.&
&
&
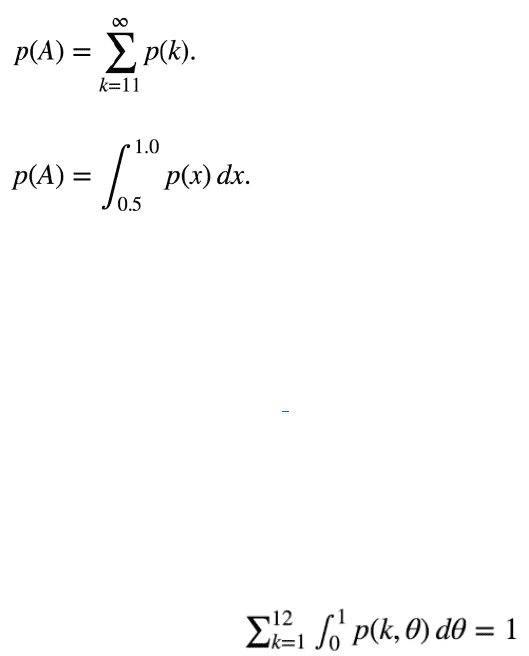
Using&the&term&“probability”&outside&of&maths&
There&are&two&main&ways&to&connect&probability&with&the&real&world:&
Bayesian&viewpoint:&
The&probability&of&an&event&represents&the&credibility&of&an&event.&
E.g.&assume&that&your°ree&of&belief&about&the&number&of&customers&at&JG’s&cafe&
tomorrow&morning&is&Bin(k|12,0.2).&If&somebody&says&there&will&be&11&customers,&then&you&
don’t&find&this&credible.&
Frequentist&viewpoint:&
The&probability&of&an&event&represents&the&frequency&of&the&event&occurring&in#the#long#run.&
E.g.&In&many&(representative)&mornings&at&JG’s,&what&is&the&fraction&of×&there&will&be&11&
customers?&
&
Bayesian&probability&
&
Bayesian¬ation&
• p()&can&mean&many&things:&a&discrete&probability#distribution&p(k),&a&
continuous&probability#density&p(x),&or&the&probability&p(A)&of&an&event.&
• Recall&the&difference&of&discrete&vs.&continuous:&Assume&the&discrete&distribution,&
and&let&A={11,12,13,…}.&Then&p(k)&is&a&probability&(represents&“mass”),&and&
&
But&in&the&continuous&distribution,&p(x)&represents&“mass&per&length”.&I.e.&if&A=[0.5,1.0],&then&
&
&
Multivariate&distributions&
If&a&sample&space&consists&of&vectors,&e.g.&(x,y)&or&(x1,&x2,&x3,&x4),&a&distribution&on&it&is&
called&multivariate.&We&will&need&such&distributions&whenever&we&have&more&than&one&
parameter.&
• Example:&
§ sample&space:1&ℕ0×[0,1]&
§ distribution:&
p(k,x)=Bin(k|12,0.2)Beta(x|0.5,2)&
• Example:&
§ same&sample&space&
§ distribution:&
p(k,θ)=Bin(k|12,θ)Beta(θ|0.5,2)&
Check&that&in&both&cases& &
&
Marginal&distributions&
• Any&bivariate&distribution&p(x,y)&on&pairs&(x,y)&admits&two&univariate&marginal&
distributions&p(x)&and&p(y).&
• Example:&
Document Summary
There is a one-to-one correspondence between probability distributions and random variables: a random variable x can take any value in the sample space, the probability that x takes a value from the set a is p(a). The two points show how any probability distribution defines a random variable x. (this is made rigorous in math5825. ) If the sample space can be written as a (possibly infinite) list, then the distribution is called discrete: Binomial distribution: x bin(n, ) and write p(x)=bin(x|n, : parameters: number n of trials; probability of success sample space: x {0,1, ,n, distribution: (note that bin(x|1, ) is also called the bernoulli distribution, and written as bern(x| ). A single fair coin toss corresponds to bern(x|0. 5). ) If it is not possible to write the sample space as a list, then the distribution is continuous. E. g. , there exists no (not even infinite) list of all real numbers between 0 and 1.