Statistical Sciences 2244A/B Chapter Notes - Chapter 26: Confidence Interval, Multiple Comparisons Problem, John Tukey
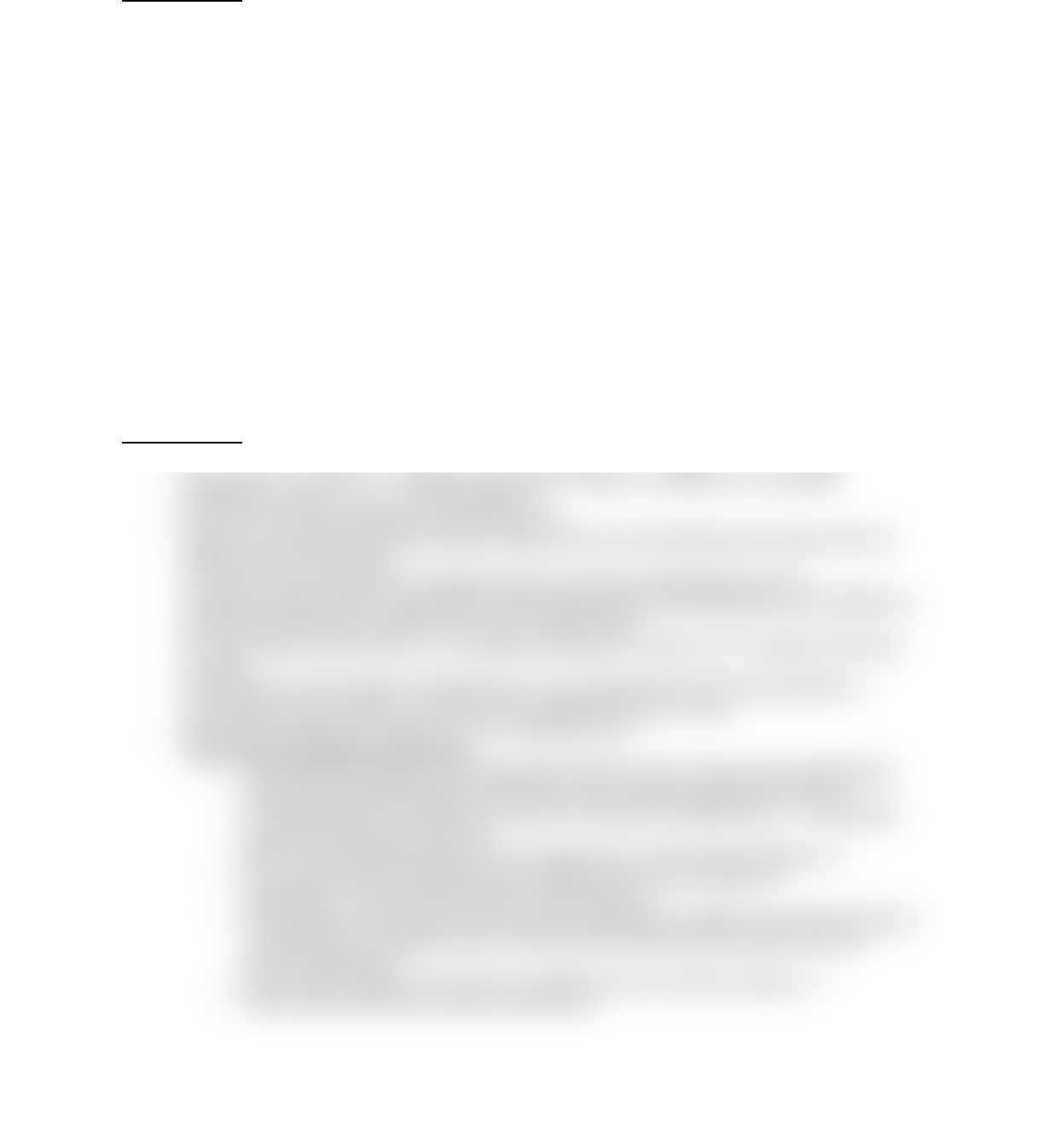
Stats 2244
Chapter 26
CHAPTER 26.1
Beyond one way ANOVA
- One-way ANOVA compares the means μ1, μ2,…, μk of k populations based on samples of
sizes n1, n2,…, nk from these populations.
- Using separate two-sample t procedures to compare many pairs of means is a bad idea, because
we don’t have a P-value or a confidence level for the complete set of comparisons together.
- This is the problem of multiple comparisons.
- One-way ANOVA gives a single test for the null hypothesis that all the population means are the
same against the alternative hypothesis that not all are the same (H0 simply is not true).
- ANOVA works by comparing how far apart the sample means are relative to the variation
among individual observations in the same sample. The test statistic is the ANOVA F statistic
- The P-value comes from an F distribution
- The required conditions for ANOVA are independent random samples from each of the k
populations (or a randomized comparative experiment with k treatments), normal
distributions for the response variable in each population, and a common standard deviation σ in
all populations.
- Fortunately, ANOVA inference is quite robust against moderate violations of the Normality and
common standard deviation conditions.
- In basic statistical practice, we combine the F test with descriptive data analysis to check the
conditions for ANOVA and to see which means appear to differ and by how much.
CHAPTER 26.2
Follow up analysis: Tukey pairwise multiple comparisons
- In general, we want to give confidence intervals for all pairwise differences among the
population means μ1, μ2,…, μk of k populations.
- We want an overall confidence level of (say) 95%.
- That is, in very many uses of the method, allthe intervals will simultaneously capture the true
differences 95% of the time.
- To do this, take the number of comparisons into account by replacing the toverall
confidence critical value t* with another critical value based on the distribution of the difference
between the largest and smallest of a set of k sample means.
- We will call this critical value m*, for multiple comparisons. Values of m* no longer come from
a t table.
- They depend on the number of populations we are comparing and on the total number of
observations in the samples, as well as on the confidence level we want.
- Use the short table of m* values for a 95% confidence level
- Tukey pairwise multiple comparisons
o In the ANOVA setting, we have independent SRSs of size ni from each of k populations
having Normal distributions with means μi and a common standard deviation σ.
o Tukey simultaneous confidence intervals for all pairwise differences μi − μj among the
population means have the form
o Here is the sample mean of the i th sample and sp is the pooled estimate of σ.
o The critical value m* depends on the confidence level C, the number of
populations k, and the total number of observations N.
o If all samples are the same size, the Tukey simultaneous confidence intervals provide an
overall level C of confidence that all the intervals simultaneously capture the true
pairwise differences.
o If the samples differ in size, the true confidence level is at least as large as C.
o That is, the conclusions are then conservative.
find more resources at oneclass.com
find more resources at oneclass.com
Document Summary
One-way anova compares the means 1, 2, , k of k populations based on samples of sizes n1, n2, , nk from these populations. we don"t have a p-value or a confidence level for the complete set of comparisons together. This is the problem of multiple comparisons. One-way anova gives a single test for the null hypothesis that all the population means are the. Using separate two-sample t procedures to compare many pairs of means is a bad idea, because same against the alternative hypothesis that not all are the same (h0 simply is not true). Anova works by comparing how far apart the sample means are relative to the variation among individual observations in the same sample. The test statistic is the anova f statistic. The p-value comes from an f distribution. Fortunately, anova inference is quite robust against moderate violations of the normality and common standard deviation conditions.