BPS 4104 Chapter Notes - Chapter 5: Position Weight Matrix, Gibbs Sampling, Prior Probability
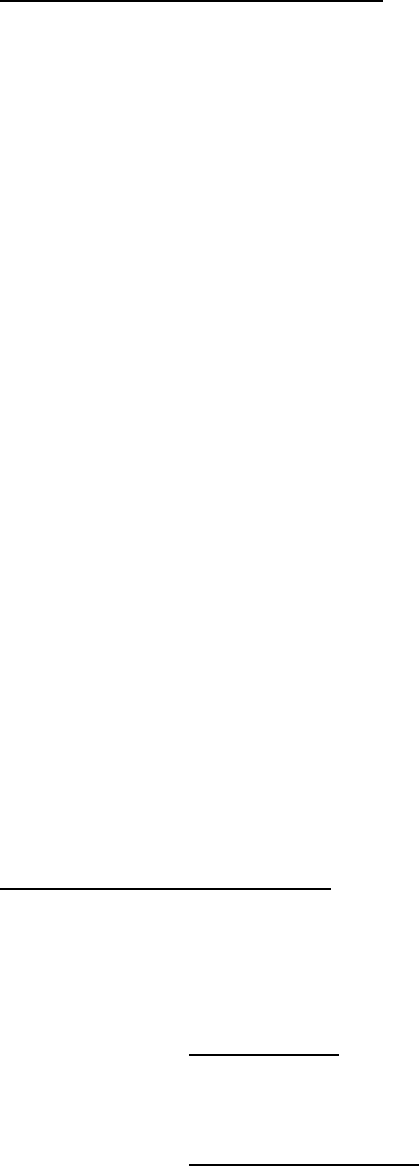
Chapter 5: Gene & Motif Prediction
-two major categories of gene & motif annotation methods
-first is based on known genes in molecular databases
-uses homology search tools (FASTA & BLAST)
-second is based on known gene structures
-is represented by GENSCAN
-most gene prediction methods are based on known sequence differences between
protein coding/non-protein coding & between motifs/non-motifs
-differences are characterized into two categories:
-signal sensors
-refers to signals with strong site dependence
-ex: knowing a nucleotide at site i improves our prediction of
nucleotide at site k
-ex: anti-Shine-Dalgarno sequence at site i in bacterial mRNA
improves prediction of presence of ATG codon 10 bases downstream
-content sensors
-does not have detectable site dependence
-nucleotide, dinucleotide or trinucleotide frequencies of sequence
may help to know whether it is coding or non-coding
-information from different sequence structures (start sites, exons, introns, etc.) &
unusual distribution of nucleotide frequencies can be used for gene finding
Bayes Theorem & Odds Ratios
-θi :designates N alternative & discrete hypotheses where i=,,…N
-Y: observation & prior probabilities associated with each hypothesis
-Bayes theorem expresses the probability of θi being true given the observed Y:
-
-theorem can be reduced when there are only two alternative hypotheses:
-
-P(θi|Y):posterior probability for hypothesis θi
find more resources at oneclass.com
find more resources at oneclass.com
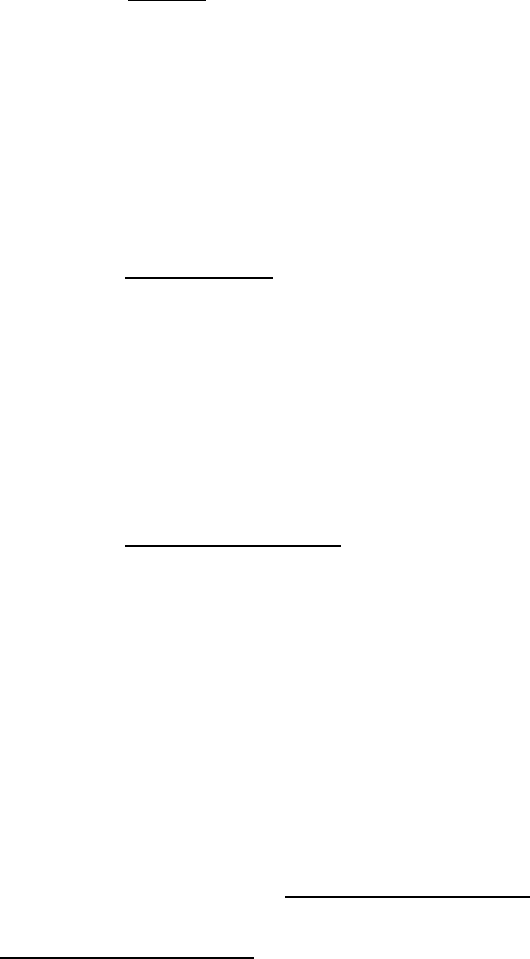
-P(Y|θi): conditional probability of having observation Y given hypothesis θi
-Pθi): the prior probability
-numerator of equation: joint probability
-denominator of equation: prior probability
-odds ratio: measures how likely a hypothesis is relative to its alternative
-the ratio of the two probabilities associated with each hypothesis
-
-Bayes theorem can also be expressed as: Ω”= Ω Ω
-example:
-bacterial genome of length L has N protein coding genes of length X1…XN
-if we randomly pick a sequence fragment of length z bases, the probability
that it is within a coding sequence is:
-
-numerator is the number of possible ways of picking the fragment of z bases
long within a coding sequence
-denominator is the number of possible ways of picking the fragment of z
bases within the genome
-z=90bp, genome length=10 000 bp, coding genes=900 & 3000
-
-probability of coding gene having an ORF at least 90 bases long=0.95
-probability of intergenic sequence having ORF at least 90 bases long=0.1
-Pθyes)=0.37554
-Pθno)=0.62446
-PY|θyes)=0.95
-P(Y|θno)=0.1
-probability that θyes is correct is:
-
Position Weight Matrix
find more resources at oneclass.com
find more resources at oneclass.com

-technique for characterizing sequence motifs from a set of aligned training
sequences
-resulting PWM can be used to scan sequence fragments and generate a score for
each sequence fragment
-large score associated with higher likelihood of fragment being one of motifs
-also essential building block of Gibbs sampler
-ex: characterize translation initiation signal in eukaryotic mRNAs
-used chromosome 22 with 508 genes, studied sequences with L of 13
-initiation signal includes initiation codon with a few bases flanking
-A4GALT ATACCATGTCCAA
-ACO2 ACAAAATGGCGCC
-ACR GGAGTATGGTTGA
-ADM2 CCGCCATGGCCCG
-first step in generating PWM: obtain site specific nucleotide frequencies
-if motif has biased nucleotide usage, prediction of motif is straightforward
-fij: designated as site specific frequency for nucleotide i(i=1,2,3,4 corresponding to
A,C,G,T) at site j(,,…
-N is the total number of sequences
-can create frequency table from this information
-
-
-given a sequence S=ACGGTACCACGTT there are two hypothesis
-1. It belongs to the 13 base translation initiation signal θyes
-. )t does not belong to base translation initiation signal θno
-sequence should share site dependence with training sequences if θyes is true
-likelihoods of observing sequence S are specified as:
-Lyes=pS|θyes)=pA1pC2pG3pG4…pT13
-Lno=pS|θno)=pA3pc4pG3pT3
-for Lno the order of sites is irrelevant
find more resources at oneclass.com
find more resources at oneclass.com
Document Summary
Two major categories of gene & motif annotation methods. First is based on known genes in molecular databases. Most gene prediction methods are based on known sequence differences between protein coding/non-protein coding & between motifs/non-motifs. Ex: knowing a nucleotide at site (cid:494)i(cid:495) improves our prediction of nucleotide at site (cid:494)k(cid:495) Ex: (cid:494)anti-shine-dalgarno(cid:495) sequence at site (cid:494)i(cid:495) in bacterial mrna improves prediction of presence of atg codon 10 bases downstream. Nucleotide, dinucleotide or trinucleotide frequencies of sequence may help to know whether it is coding or non-coding. Information from different sequence structures (start sites, exons, introns, etc. ) unusual distribution of nucleotide frequencies can be used for gene finding. I :designates n alternative & discrete hypotheses (cid:523)where i=(cid:883),(cid:884), n(cid:524) Bayes theorem expresses the probability of i being true given the observed y: Y: observation & prior probabilities associated with each hypothesis. Theorem can be reduced when there are only two alternative hypotheses: