COMMERCE 2QA3 Study Guide - Final Guide: Dependent And Independent Variables, Standard Deviation, The Intercept
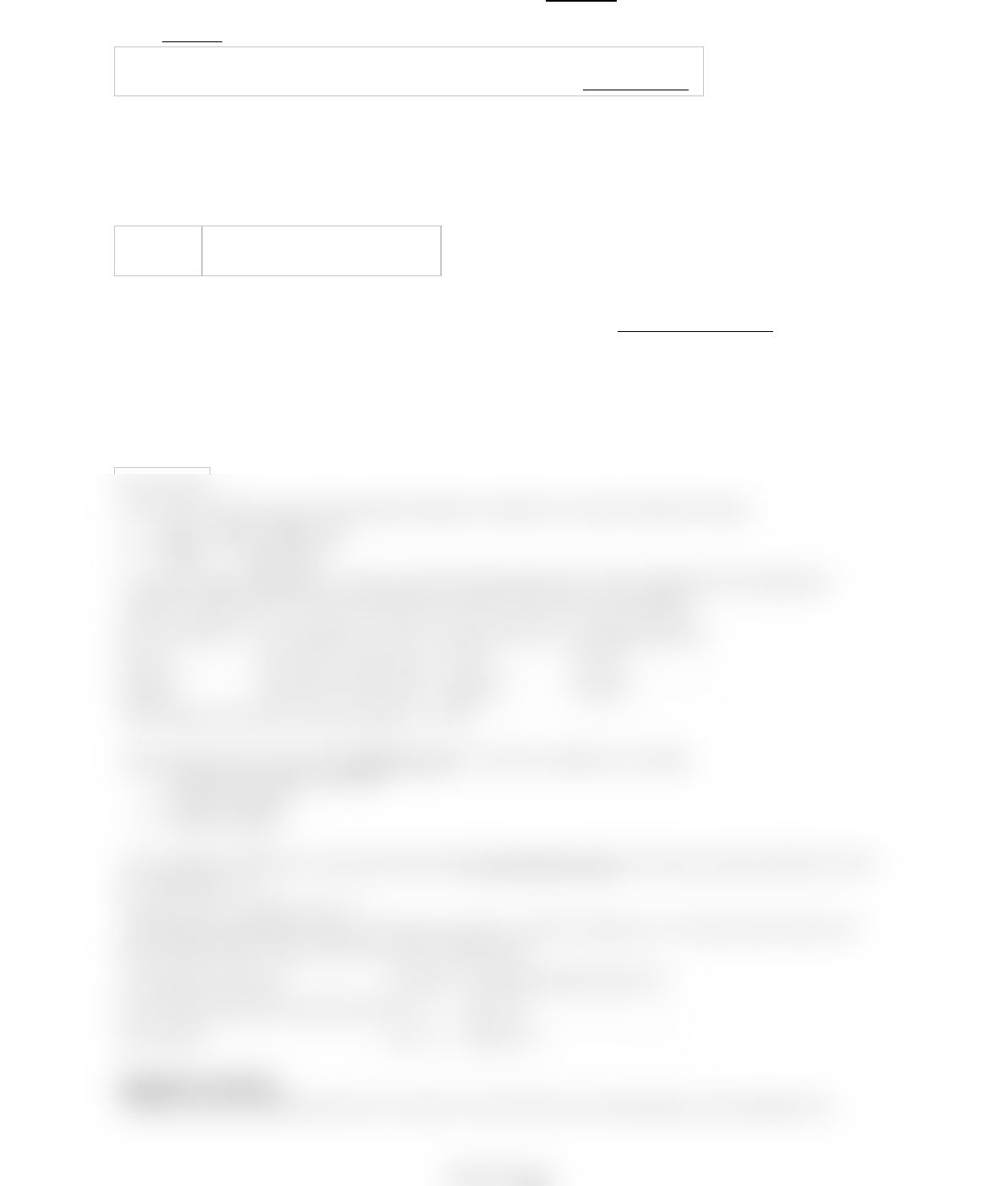
Linear Model
Straight line summarizing general pattern in a scatterplot. The regression line is one that best fits all the
points - predicts the values of the dependent variable for values of an independent variable. There are always
some residuals (errors), unless all the points form a perfect line.
Where and are estimated numbers from the data and
is the predicted value.
Residual
The difference between the predicted and actual dependent variable value, denoted by . They are part of the
data that has not been modelled and determine if the model makes sense. In a scatterplot, the residuals
against predicted values should show no patterns, directions, or shapes. If nonlinearities, outliers, or clusters in
the residuals are seen, then you must determine what the regression model is missing.
= predicted dependent variable
= actual dependent variable
The standard deviation of the residuals is
and should fulfill the equal spread condition, where the
standard deviation around the line is the same wherever it is applied.
Some residuals will be positive and some negative, so to get a good assessment of how the line fits the data,
we take the -
Line of Best Fit/Least Squares Line
The line for which the sum of the squared residuals is smallest. is used to denote the slope.
is the correlation coefficient, and are the standard deviations of the independent and dependent
variables, respectively. The units of the slope are a ratio of the units of the variables.
If the correlation is
The scatterplot runs from
Slope of the line is
Standard deviation
Positive
Lower left to upper right
Positive
Positive
Negative
Upper left to lower right
Negative
Positive
The intercept of the line is found using
.
Quantitative variables condition
1.
Linearity condition
2.
Outlier condition
3.
Least squares lines are also called regression lines. To check for regression, evaluate
The correlation coefficient can be expressed with the standardized value. They have simple properties in that
and the .
Thus,
and .
If working with standardized values, the slope is equal to and the intercept is . The formula for that line of
best fit becomes
. From this, we can conclude that:
x compared to the mean
Formula
Predicted standard value of y
One standard deviation over the mean
Equal to r
At the mean
Equal to 0
Regression to the Mean
Indicates that each predicted value of y is closer to its mean than its corresponding x value. Between two
variables, there is only one correlation coefficient . There are two regression lines: one has x as the
Introduction to Linear Regression
September 14, 2017
4:53 PM
Statistics Page 1