PSYC2009 Study Guide - Final Guide: Squared Deviations From The Mean, Analysis Of Variance, Null Hypothesis
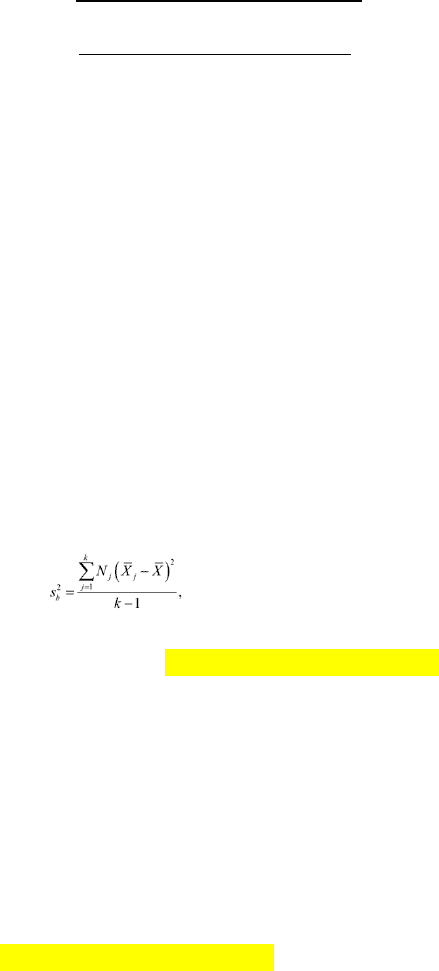
Analysis of Variance (ANOVA)
Rationale for ANOVA
• In a situation where we simply want to find out whether any means differ from each other, we
use the F test to judge whether differences among means could have happened by chance or
not.
• We do this by partitioning the sums of the squared deviations of each score from the grand
mean, into two parts: SSb and SSw.
o SSb - the sum of squares between groups, which is the sum of the squared deviations
of each group's mean from the overall "grand" mean.
o SSw - the sums of squares within groups, which is the sum of the squared deviations of
each case from its group mean.
Assumptions of ANOVA
• At least interval-level measurement for dependent variable.
• The dependent variable has a Normal distribution.
• Homogeneity of variance: the population variances of the groups are all equal to one another,
i.e. the independent variable affects only the means, not the variances.
• H0: The groups means are all equal.
Partitioning the Variance
• ANOVA begins by partitioning the total variance of the data into two components.
1. The pooled variance estimates, s2pooled which is an estimate of the population
variance of the scores, σ2.
o In ANOVA, s2pooled is usually denoted by s2w, where the w stands for 'within
experimental conditions'. It is based on SSw.
2. The sample estimate of the variance between experimental conditions is denoted
by sb2, and it also is an estimate of the population variance, σ2. It is based on SSb.
• SSb treats the means of each group as if they are raw scores and plugs them into the formula
for a sample variance. Since there are k experimental conditions, we divide by k-1. Our
estimate is
where Nj is the number of cases in the jth experimental condition.
• ANOVA uses an F statistic: F(dfb, dfw) = (s2b/ s2w)/(σ2b/σ2w) to compare the variance between
experimental conditions against the variance of scores within conditions.
• Its sampling distribution is an F distribution with dfb = k-1 and dfw = N-k.
• The corresponding F test associated with ANOVA has a null that σ2b = σ2w = σ2, which is
equivalent to saying σ2b/σ2w = 1.
• If the means' variability is influenced only by sampling error, then s2b and s2w should both be
σ2.
• If, on the other hand, the means' variability is also influenced by experimental effects, then
then s2b > s2w.
• Another way to think of this is that s2b is determined by experimental effects + sampling error,
whereas s2w is determined by sampling error alone.
• F-ratio: s2b /s2w = (effects + error)/error
• If a value is found to be plausible, this raises two possibilities:
1. There is no effect from age on reading-scores.
2. The study lacks sufficient power to detect this effect.
• Having failed to reject a null hypothesis, when would it make sense for researchers to think
that it's because the study was underpowered, and when would it make sense for them to
think that there probably is no effect?
find more resources at oneclass.com
find more resources at oneclass.com
Document Summary
Partitioning the variance: anova begins by partitioning the total variance of the data into two components, the pooled variance estimates, s2 pooled which is an estimate of the population variance of the scores, 2. It is based on ssw. pooled is usually denoted by s2 w, where the w stands for "within by sb: the sample estimate of the variance between experimental conditions is denoted. 2, and it also is an estimate of the population variance, 2. Ssb treats the means of each group as if they are raw scores and plugs them into the formula for a sample variance. Since there are k experimental conditions, we divide by k-1. Its sampling distribution is an f distribution with dfb = k-1 and dfw = n-k. = 2: the corresponding f test associated with anova has a null that 2 w = 2, which is b/ 2 w = 1. equivalent to saying 2.