PSYC2009 Study Guide - Final Guide: Statistical Inference, Simple Random Sample, Sampling Frame
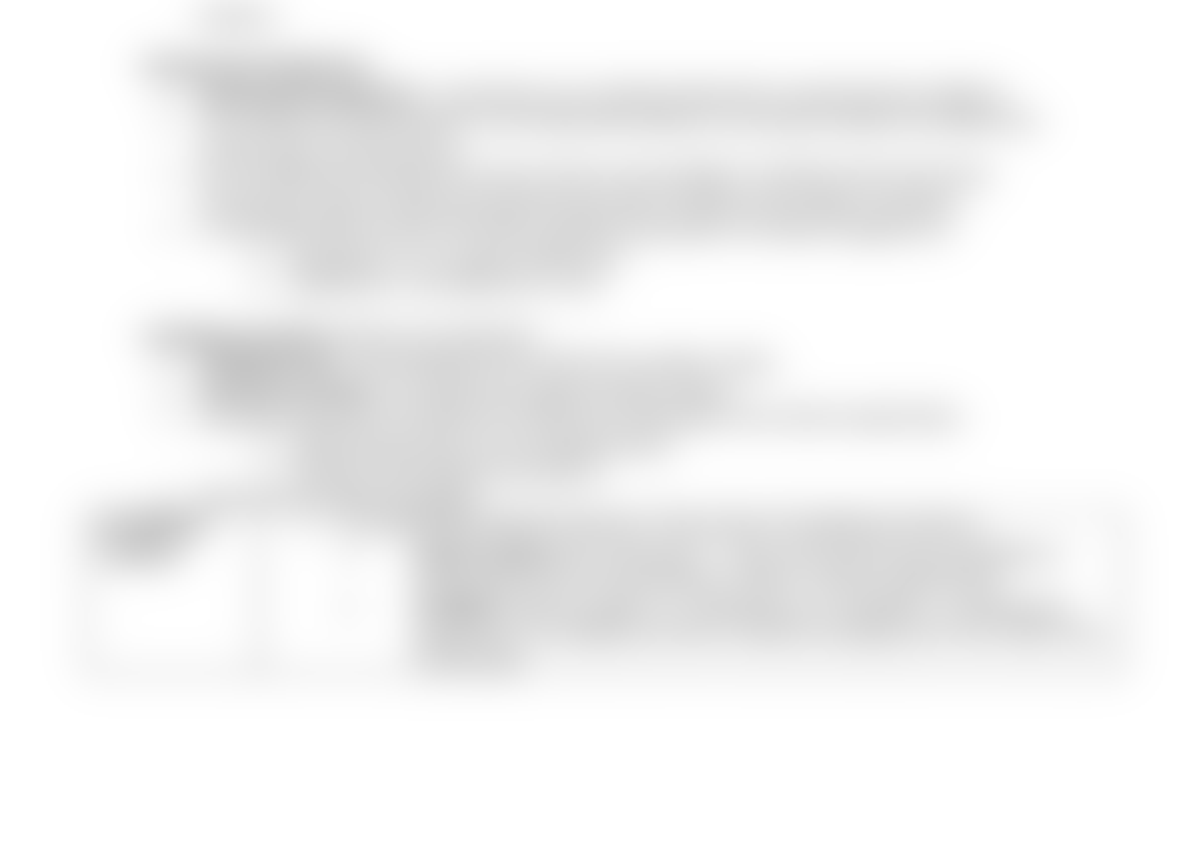
Introduction to Inferential Statistics
Motives for using inferential statistics
Sampling
• Population - all individuals or objects of interest → population parameter (the actual %).
• Sample - a subset of the population → sample estimate.
Questions that inferential statistics can answer about sampling:
• What kind of sample should you get?
• How do you select such a sample and avoid bias?
• How big should the sample be?
• How close is your estimate likely to be to the population percentage?
• What degree of confidence should you have in this estimate?
Inferential statistics can give inferences about what?
• Most studies involve generalisations and inferences beyond the people actually studied.
Among the kinds of generalisation made are: to the population from which the sample came;,
across time or to other populations.
• Usually only the first kind is covered by inferential statistics.
Diagnosis
• Eg: A head-trauma patient's short-term memory scores 2 standard deviations below the
population mean for a test that has been normed on the adult population.
• Has his short-term memory been damaged by the head-trauma, or is it likely that he just
happens to have a rather poor short-term memory?
• Could his test score have come from the normal adult population of scores, or is it from
another (memory-damaged) population?
• The clinician can answer this question by using inferential statistics.
• Can take the variability surrounding the mean and SD into account by using inferential
statistics.
Randomised Assignment
• Randomised assignment – participants are randomly allocated to experimental conditions.
• This enables the experimenter to use inferential statistics to ascertain whether the differences
may be real or just by chance.
• Eg: The difference between the mean of how much gamblers would bet with real money,
compared to tokens. Results showed that the token condition were higher risk takers.
• It is possible that by chance the token condition got greater risk-takers assigned to it.
1. Hypothesis 1: it’s a chance difference;
2. Hypothesis 2: the difference is real.
Sampling procedures all have two elements:
• Sampling frame - the population from which the sample is taken.
• Selection procedure - by which the sample actually is taken.
• Desirable properties of samples for inference to population from which sample taken:
1. Representativeness in the sampling frame,
2. No bias in the selection procedure.
• There are two kinds of samples:
Probabilistic
(random)
• For inferential statistical purposes. Three types of sampling procedures:
1. Simple random and "systematic" – each unit has the same probability of
being selected from the population. With or without replacement.
2. Stratified random sample, i.e. stratification on variable(s) – stratifying the
population on variable(s) and then randomly sampling units from within each
of the strata.
find more resources at oneclass.com
find more resources at oneclass.com
Document Summary
Sampling: population - all individuals or objects of interest population parameter (the actual %). Sample - a subset of the population sample estimate. Inferential statistics can give inferences about what: most studies involve generalisations and inferences beyond the people actually studied. Among the kinds of generalisation made are: to the population from which the sample came;, across time or to other populations: usually only the first kind is covered by inferential statistics. Results showed that the token condition were higher risk takers. It is possible that by chance the token condition got greater risk-takers assigned to it: hypothesis 1: it"s a chance difference, hypothesis 2: the difference is real. Sampling frame - the population from which the sample is taken. Simple random and systematic each unit has the same probability of being selected from the population. Stratified random sample, i. e. stratification on variable(s) stratifying the population on variable(s) and then randomly sampling units from within each of the strata.