PSY 305 Lecture Notes - Lecture 6: Prosopagnosia, Hemispatial Neglect, Superior Temporal Sulcus
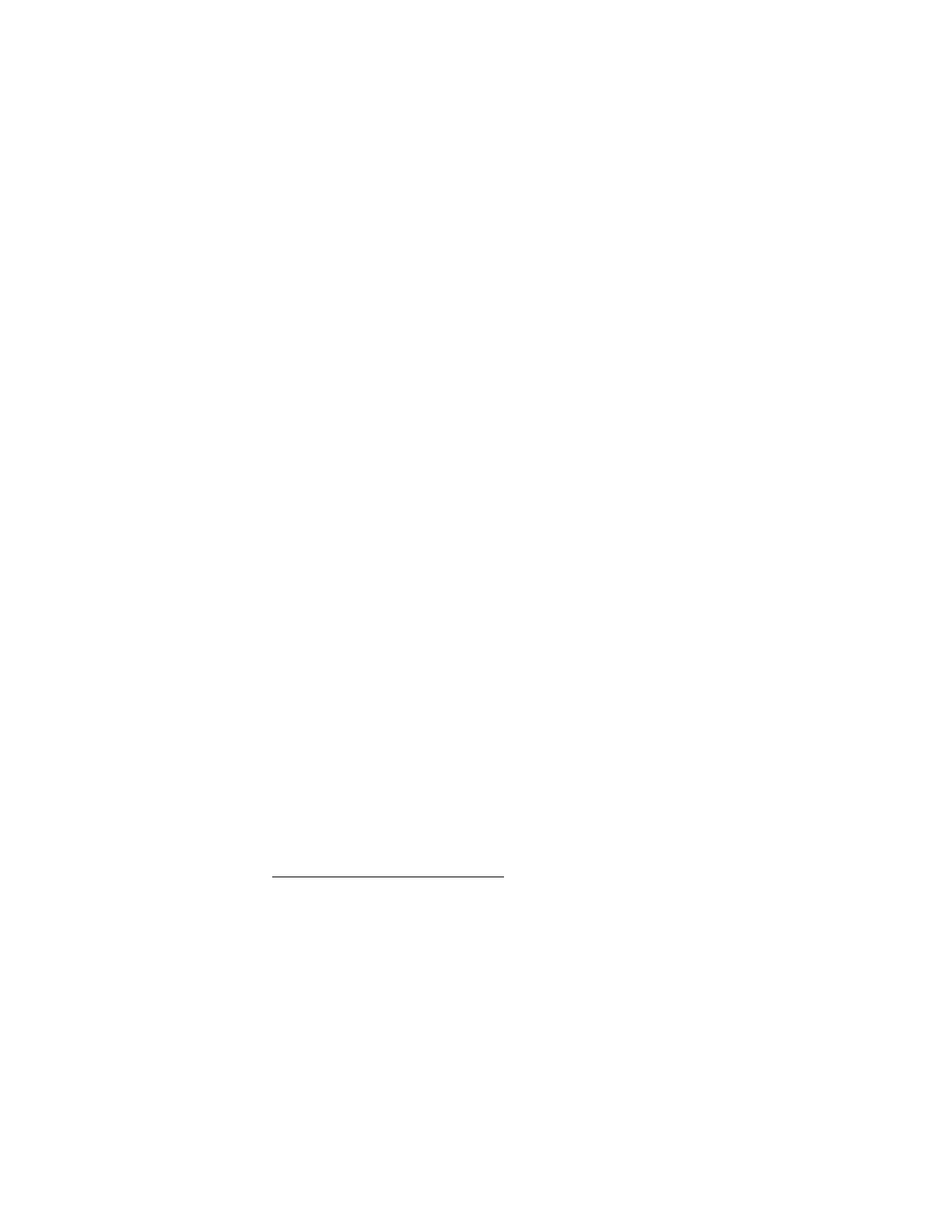
Psychology 305 Notes
12 October 2017
Feature nets
● Everything is broken down by features and feature detectors, simple detections of light
● The feature detectors first pick up edges / shapes
○ Each particular detector likes a particular shape
● Next, letter detectors add up those shapes
○ You start to see a strengthening of connections between letter detectors and
feature detectors. Start to recognize things bc “this input happened before” → “oh
i’ve seen this before”
● Next, the word detector layer adds up that input, how we get words
○ Connections are also strengthened with time, we start to recognize a certain
word a certain set of words
● What about confusions?
○ Network can be primed to be aware of a particular set of inputs
○ We think we are seeing these
● The bigram layer helps the system recover from confusion about individual letters
○ Here, only some letter “O” features were detected, but this is compensated for by
higher baseline activity of the “CO” detector
● Also explains errors of over-regularization
○ Here, the presented stimulus is “CQRN” but is likely to be misread as “CORN”
○ However, the network’s biases usually help achieve correct perception
Feature Nets Cont
● McClelland and Rumelhart’s (1981) model of word recognition included two additions
○ Excitatory and inhibitory connections between detectors
○ Top-down connections from words to letters and letters to features
● A much more complex feature net with feedforward and feedback loops with
hierarchical detectors!
● More like the brain!!
Object Recognition Models
● Models of object recognition differ on whether object recognition depends on viewpoint
○ In the recognition components model, geons result in viewpoint-independent
recognition
○ Other proposals are viewpoint-dependent, requiring the remembered
representation to be “rotated” onto alignment with the current view
Object Recognition from Different Viewpoints
● Structural-description models
○ 3-D objects are based on 3-D volumes called volumetric features that are
combined for a given shape
○Marr’s model proposed a sequence of events using simple geometrical features
○ The sequence begins with identifying edges and proceeds to recognition of the
object
Document Summary
Everything is broken down by features and feature detectors, simple detections of light. The feature detectors first pick up edges / shapes. Each particular detector likes a particular shape. Next, letter detectors add up those shapes. You start to see a strengthening of connections between letter detectors and feature detectors. Start to recognize things bc this input happened before oh i"ve seen this before . Next, the word detector layer adds up that input, how we get words. Connections are also strengthened with time, we start to recognize a certain word a certain set of words. Network can be primed to be aware of a particular set of inputs. The bigram layer helps the system recover from confusion about individual letters. Here, only some letter o features were detected, but this is compensated for by higher baseline activity of the co detector. Here, the presented stimulus is cqrn but is likely to be misread as corn .