QBUS3820 Lecture Notes - Lecture 2: Exploratory Data Analysis, Linear Algebra, E.G. Time
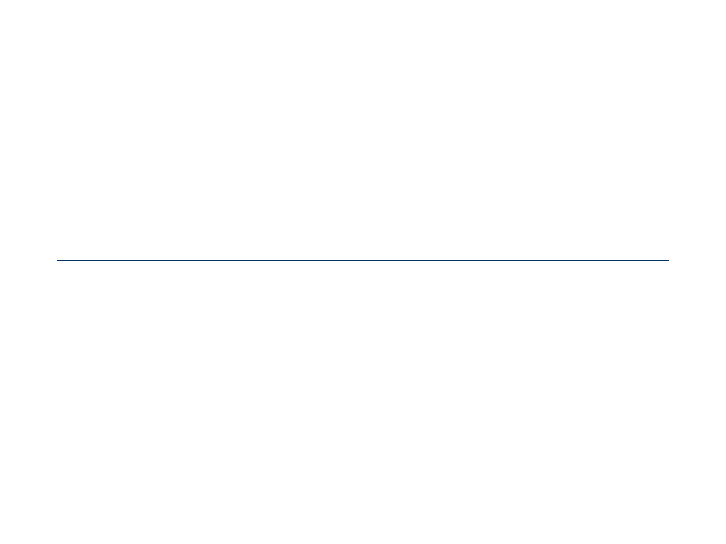
Machine Learning and Data Mining in
Business
Lecture 2: Linear Regression
Associate Professor Peter Radchenko
Semester 1, 2018
Discipline of Business Analytics, The University of Sydney Business School
find more resources at oneclass.com
find more resources at oneclass.com
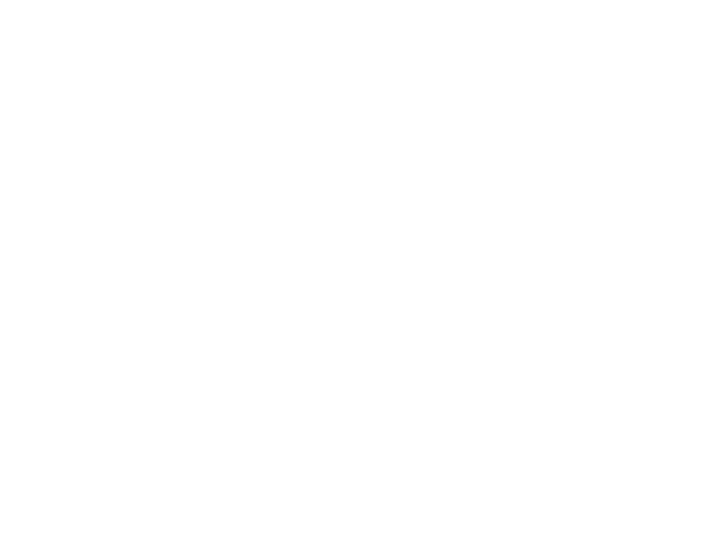
Lecture 2: Linear Regression
1. Introduction
2. The least squares method
3. Interpreting a linear regression model
4. EDA and residual diagnostics
5. Data transformation
6. Categorical predictors
7. Polynomial regression
2
find more resources at oneclass.com
find more resources at oneclass.com
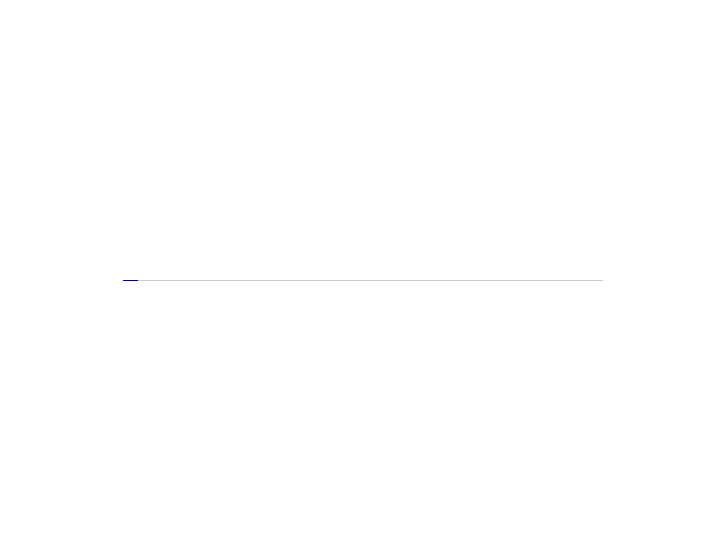
Introduction
find more resources at oneclass.com
find more resources at oneclass.com
Document Summary
Discipline of business analytics, the university of sydney business school. Lecture 2: linear regression: introduction, the least squares method, interpreting a linear regression model, eda and residual diagnostics, data transformation, categorical predictors, polynomial regression. Linear regression is a simple and widely used method for supervised learning. There are several important reasons for developing an in-depth understanding of this method: it is extremely useful conceptually. Business problem: predicting customer purchasing behaviour, with the objective of targeting sales e orts. Response: amount spent (dollars) on direct marketing products. Predictors: customer salary, age group, gender, marital status, number of children, number of catalogs sent to, spending history (high/low/medium), whether customer is a homeowner, location of nearest physical store (far/close). Computing the correlations for the training data reveals that the customer salary is the predictor with strongest linear relationship with the response. For example, we can consider the following model for predicting the amount spent by a customer: