QBUS3820 Lecture Notes - Lecture 1: Software Engineering, No Free Lunch Theorem, Unsupervised Learning
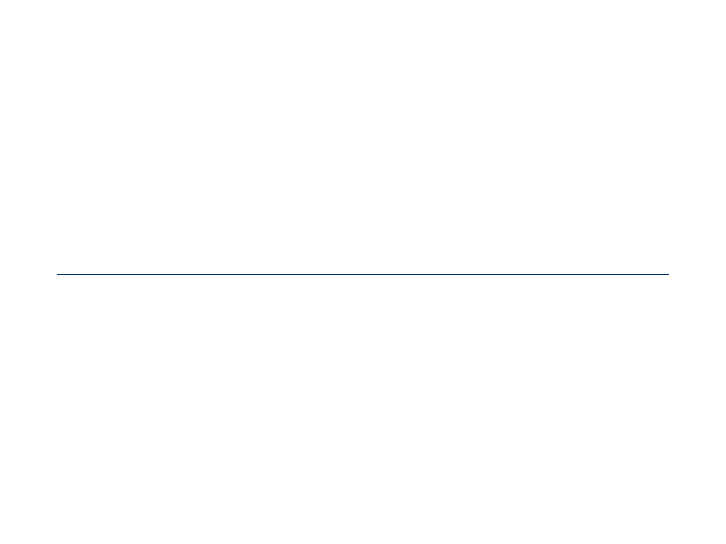
QBUS3820: Machine Learning and Data
Mining in Business
Lecture 1: Introduction
Associate Prof. Peter Radchenko
Semester 1, 2018
Discipline of Business Analytics, The University of Sydney Business School
find more resources at oneclass.com
find more resources at oneclass.com
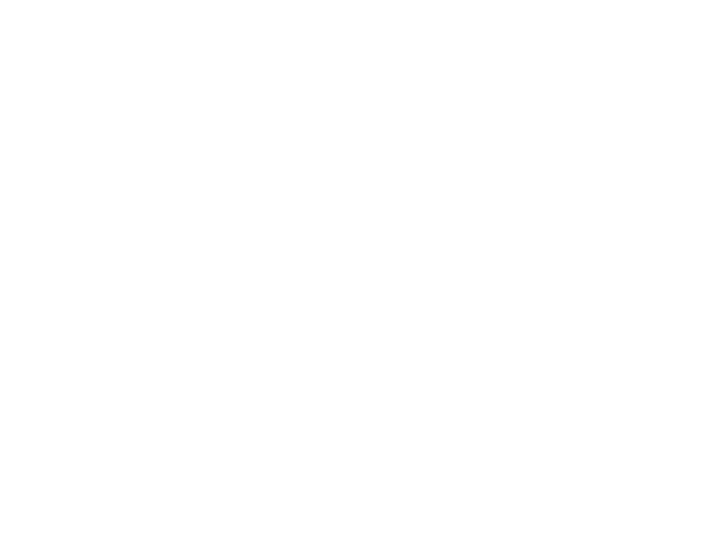
Teaching Team
•Unit Coordinator and Lecturer:
Associate Prof. Peter Radchenko
•Tutor:
Ransalu Senanayake
•Python Helper:
Kelvin Hsu
2
find more resources at oneclass.com
find more resources at oneclass.com
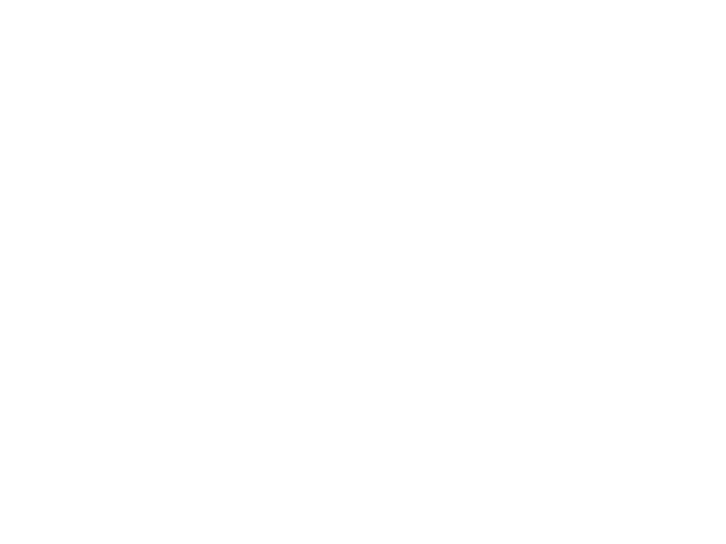
Lecture 1: Introduction to Machine Learning & Data Mining
1. Introduction
2. Business Examples
3. Notation
4. Statistical decision theory
5. Evaluating model performance
6. Overview of some key concepts and themes
3
find more resources at oneclass.com
find more resources at oneclass.com
Document Summary
Discipline of business analytics, the university of sydney business school. Lecture 1: introduction to machine learning & data mining: introduction, business examples, notation, statistical decision theory, evaluating model performance, overview of some key concepts and themes. Machine learning is a set of methods for automatically detecting patterns in data and using them for predicting future data and guiding decision making. We can think of statistical learning as a framework for machine learning that draws on statistics. Two trends bring statistical learning to the forefront of successful business decision making: we are in the era of big data. There are two main types of learning: in predictive or supervised learning, the objective is to learn a function to predict an output variable y based on observed input variables x1, . , xp: in descriptive or unsupervised learning, we only have inputs, x1, . , xp, and the goal is to nd interesting patterns in this data.