PUBH2007 Lecture Notes - Lecture 3: Prostate Cancer Screening, Random Assignment, Cluster Sampling
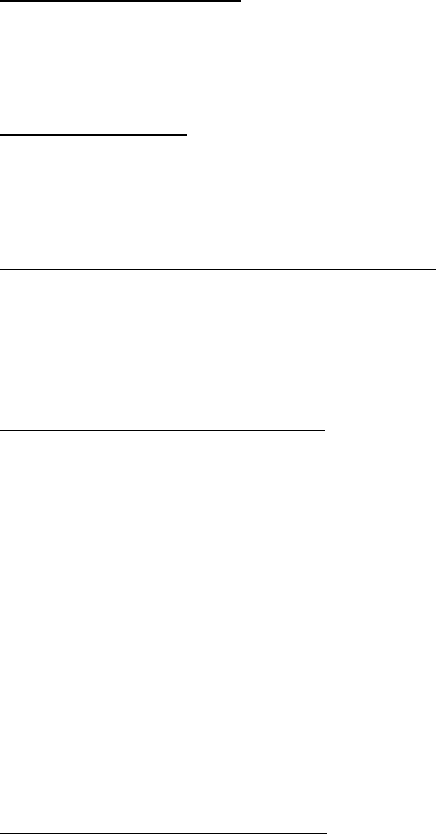
PUBH2007 LECTURE THREE
This week:
• Reasons for sampling
• Different methods of sampling
• Design of experiments and other types of studies
Last two weeks: exploratory data analysis
Now: statistical inference
Exploratory Data Analysis:
• Purpose: unrestricted exploration of the data, searching for interesting patterns
• Conclusions apply only to the individuals and circumstances for which we have data
• Conclusions: informal, based on what we see in the data
Statistical inference:
• Purpose: to answer specific questions, posed before the data were produced
• Conclusions apply to a larger group of individuals or broader class of circumstances
• Conclusions: formal, backed by a statement of our confidence in them
Populations and samples: direction of inference
Population (happy and sad) -> Sampling -> Sample (all happy)
• Need to express uncertainty about the assumptions we make i.e. confidence intervals
Populations and Samples - example
Population we want information about - entire group of interest e.g. everyone in Australia with asthma
(target population)
Population actually studied - subgroup of target population available for study e.g. people obtaining
asthma medication in pharmacies
Sample selected - people selected for study e.g. people obtaining asthma medication from 20 selected
pharmacies in Brisbane city
Sample on whom data are collected - e.g. people obtaining asthma medication from these 20 selected
pharmacies in Brisbane City on 9th August 2016
Population and Sample - definitions
The distinction between population and sample is basic to statistics. To make sense of any sample result,
you must know what population the sample represents.
find more resources at oneclass.com
find more resources at oneclass.com
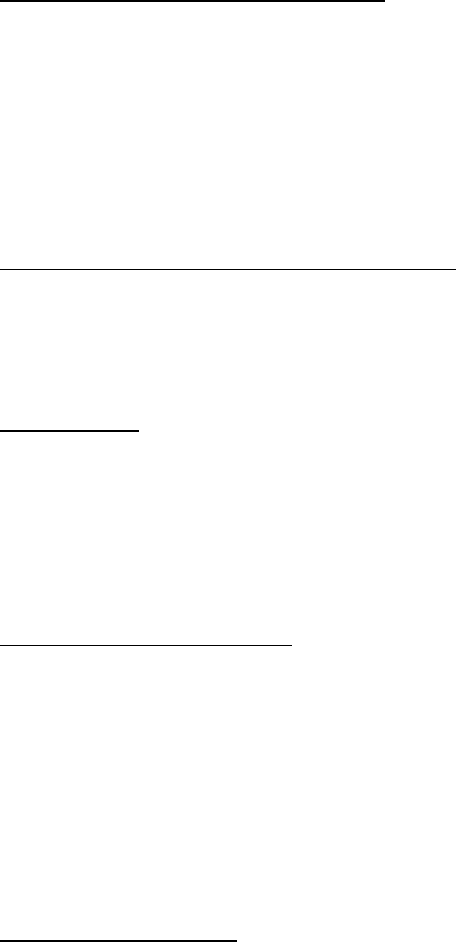
Population: in a statistical study; the entire group of individuals about which we want information
Sample: the part of the population from which we actually collect information. We use information from
a sample to draw conclusions about the entire population
Sampling design: describes exactly how to choose the sample from the population
Non-random methods of sample selection
Volunteers : e.g. SMS votes for TV show are likely to be biased by people who hold strong views, certain
age groups
Convenience sample: e.g. 'intercept' sample in shopping centre - liable to bias - who is in the shopping
centre at the particular time? Near which shops?
Haphazard sample: selected by some process that does not use random numbers
Non-random methods of sample selection - issues
1. Usually we do’t kow who the actual target population are exactly
2. Hence it is difficult to assess true extent of biases
3. Sampling method may not be reproducible
Randomisation
• Idea due to R. A. (Sir Ronald) Fisher
• Use impersonal CHANCE to assign participants to groups
E.g. tossing coin/drawing name from hat
• We use a table of random numbers to illustrate the idea
• In practice use a computer to generate the random numbers
SIMPLE RANDOM SAMPLE (SRS): of size n consists of n individuals from the population chosen in such a
way that every set of n individuals has an equal chance to be the sample actually selected
• Assign study ID numbers to 12 study participants
• Group 1 and Group 2
• Take pairs of numbers (because we want values between 01 and 12)
• Discard 11, those greater than 12, and any already selected
• Continue generating random numbers in random number table until you have 6 people in Group 1
(based on random number table)
• Assign those that have not been selected for Group 1 to Group 2
Table of random numbers
• Digits , , …. 9 ae listed so that the digit i ay positio has a eual hae of eig , ….9
• Digits in different positions are independent, i.e. value in one doesn't influence value in any other
• Any pair of numbers has an equal chance of being 0, ….99
find more resources at oneclass.com
find more resources at oneclass.com
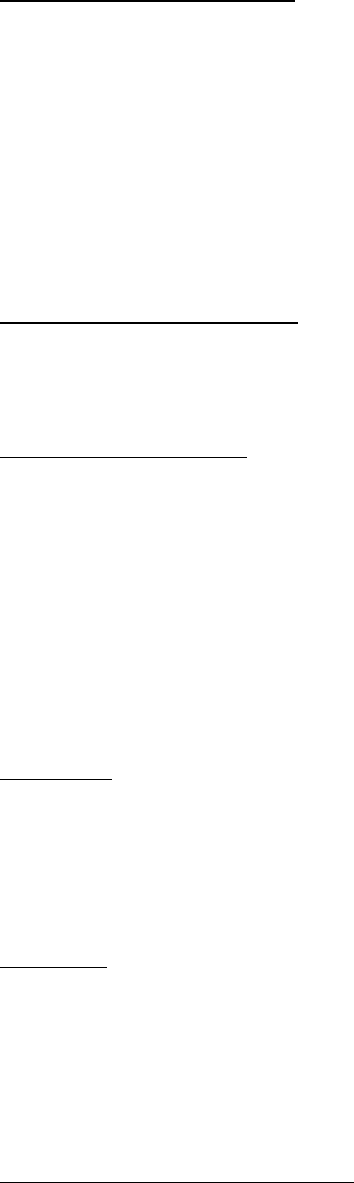
• Ay tiple of ues has a eual hae of eig , ….999
Advantages of random selection
• Can be used for choosing a sample of participants for a survey or for allocating participants to
groups for a comparative study
• Uses chance to select participants or allocate participants to groups
• Prevents bias or systematic favouritism
• Creates groups which are, on average, similar before any intervention is applied, so interpretation of
the study findings is clearer
• Can be done efficiently by computer (much better than similar example above) which can cope with
much more complex designs
• Easy to explain and reproducible
• Minimises bias (provided you can collect valid data form every participant selected in the sample)
Simple random sampling - issues
• You need a SAMPLE FRAME i.e. list of target population - often very difficult to obtain
• Impractical in many situations
Observation vs Experiment
Observational study: we observe individuals and measure variables of interest but do not attempt to
influence the responses. The purpose is to describe some group or situation.
Experiment: deliberately impose some treatment on individuals to measure their responses. The
purpose is to study whether the treatment causes a change in the response.
When the goal is to understand cause and effect, experiments are the only source of fully convincing
data. But results may not be generalisable to all situations.
Case report:
• Unusual observation about a single case with wider implications
• E.g. adverse reaction to treatment, strange signs/symptoms for known condition, new disease
• Can alert other people to look for other cases or causes
• OBSERVATIONAL STUDY
Case series:
• Data from a number of cases (e.g. breast cancer cases among women at ABC studio in Toowong)
• Usually there isn't a clear testable research question
• May be biases, unrepresentative or not generalisable to other settings
• May lead to formulation of testable hypothesis for further studies
• OBSERVATIONAL STUDY
Cross-sectional study or sample survey
find more resources at oneclass.com
find more resources at oneclass.com
Document Summary
This week: reasons for sampling, different methods of sampling, design of experiments and other types of studies. Exploratory data analysis: purpose: unrestricted exploration of the data, searching for interesting patterns, conclusions apply only to the individuals and circumstances for which we have data, conclusions: informal, based on what we see in the data. Statistical inference: purpose: to answer specific questions, posed before the data were produced, conclusions apply to a larger group of individuals or broader class of circumstances, conclusions: formal, backed by a statement of our confidence in them. Population (happy and sad) -> sampling -> sample (all happy: need to express uncertainty about the assumptions we make i. e. confidence intervals. Population we want information about - entire group of interest e. g. everyone in australia with asthma (target population) Population actually studied - subgroup of target population available for study e. g. people obtaining asthma medication in pharmacies.